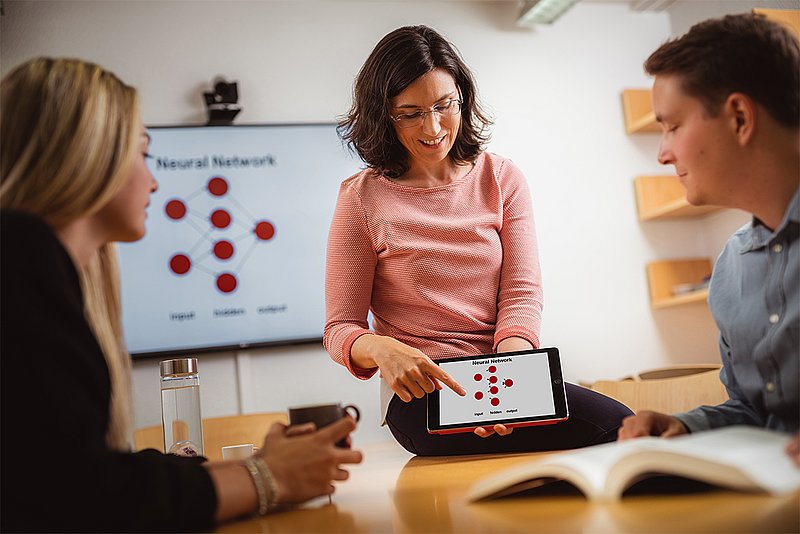
Research fields
The Laboratory for Web Science actively researches in the research fields of GeoHealth Analytics and Data Science for Energy, Environment and Materials.
The Laboratory for Web Science (LWS) conducts application-oriented research fields of data science, which is complemented by our service offering, mainly implementing and prototyping solutions for industry and service partners.
The interdisciplinary team consists of scientists from different backgrounds, including physics, mathematics and computer science. Our goal is to facilitate innovation and technology transfer through close cooperation with industry as well as national and international research partners.
The LWS has been a member of the Swiss Alliance for Data-Intensive Services since 2017. The aim of the Alliance is to support companies in the development of new products and services through cooperation and research partners.
The LWS team is strongly represented in teaching - from fundamentals to advanced topics. in the field of Data Science. The results of the LWS research flow into the teaching.
The term data science covers a wide range of individual aspects and tasks, including data selection and linking, analytics, technical implementation, corporate relevance and ethical issues.
Information and knowledge are the raw materials in every company which, if used correctly, can offer a decisive economic advantage. We distinguish between structured data, which are stored in a defined and fixed form (e.g. in databases and tables), unstructured data (data, which are available in all possible formats and platforms, e.g. e-mails, audio and video files etc.), and semi-structured data, e.g. HTML and XML.
Due to the development of information technology, ever larger amounts of data are available. These often contain implicit knowledge which, if known, would have great economic or scientific significance. Data mining is an area of research that deals with the search for potentially useful knowledge in large amounts of data, and machine learning is one of the key technologies within this area.
The aim of machine learning is to develop methods for the implementation of adaptive technical systems. Machine learning can be divided into two large classes: In supervised learning, systems for the classification and modelling of functional dependencies are trained based on existing example data. The goal of unsupervised learning is to autonomously find relevant structures in data.
The LWS evaluates internal and external data using machine learning methods.
Deep learning as a subset of machine learning opens up further undreamt-of possibilities in dealing with data. These are neural networks with many layers between data input and output. These deep networks enable the mapping of classification functions with high non-linearity, i.e. very complex tasks can be solved. The best example are convolutional neural nets, which as a disruptive technology have led to enormous progress in the field of image and signal processing.
The LWS has built up a lot of know-how and uses this technology for projects.
The Laboratory for Web Science actively researches in the research fields of GeoHealth Analytics and Data Science for Energy, Environment and Materials.
We are pleased to present you a selection of our projects in the field of research with "Data Science" and "GeoHealth Analytics".
Below you will find a selection of publications and presentations of the Laboratory for Web Science (LWS).
We would be pleased to introduce our research team to you.